序号 | 论文题目 | 作者 | 单位 | 摘要 | 关键词 | 论文链接 |
---|---|---|---|---|---|---|
1 | MIMO-SPEECH: END-TO-END MULTI-CHANNEL MULTI-SPEAKER SPEECH RECOGNITION | Xuankai Chang1,2, Wangyou Zhang2, Yanmin Qian2†, Jonathan Le Roux3, Shinji Watanabe1† | 1Center for Language and Speech Processing, Johns Hopkins University, USA 2SpeechLab, Department of Computer Science and Engineering, Shanghai Jiao Tong University, China 3Mitsubishi Electric Research Laboratories (MERL), USA | MIMO-Speech, which extends the original seq2seq to deal with multi-channel input and multi-channel output so that it can fully model multi-channel multi-speaker speech separation and recognition. MIMO-Speech is a fully neural end-to- end framework, which is optimized only via an ASR criterion. It is comprised of: 1) a monaural masking network, 2) a multi-source neural beamformer, and 3) a multi-output speech recognition model. | Overlapped speech recognition, end-to-end, neural beamforming, speech separation, curriculum learning. | https://arxiv.org/pdf/1910.06522.pdf |
2 | IMPROVING MANDARIN END-TO-END SPEECH SYNTHESIS BY SELF-ATTENTION AND LEARNABLE GAUSSIAN BIAS | Fengyu Yang1, Shan Yang1, Pengcheng Zhu2, Pengju Yan2, *Lei Xie*1∗ | 1Shaanxi Provincial Key Laboratory of Speech and Image Information Processing, School of Computer Science, Northwestern Polytechnical University, Xian, China 2Tongdun AI Lab | We introduce a novel self-attention based encoder with learnable Gaussian bias in Tacotron. The proposed approach has the ability to generate stable and natural speech with minimum language-dependent front-end modules. | Tacotron, end-to-end, speech synthesis, self-attention, Gaussian bias | http://lxie.nwpu-aslp.org/papers/2019ASRU_YFY.pdf |
3 | LEARNING HIERARCHICAL REPRESENTATIONS FOR EXPRESSIVE SPEAKING STYLE IN END-TO-END SPEECH SYNTHESIS | Xiaochun An1†, Yuxuan Wang2, Shan Yang1,2, Zejun Ma2, *Lei Xie*1⇤ | 1Shaanxi Provincial Key Laboratory of Speech and Image Information Processing, School of Computer Science, Northwestern Polytechnical University, Xi’an, China 2 ByteDance AI Lab | we introduce a hierarchical GST archi- tecture with residuals to Tacotron, which learns multiple-level disentangled representations to model and control different style granularities in synthesized speech. | Speaking style, disentangled representations, hierarchical GST, style transfer | http://lxie.npu-aslp.org/papers/2019ASRU_AXC.pdf |
4 | BOOTSTRAPPING NON-PARALLEL VOICE CONVERSION FROM SPEAKER-ADAPTIVE TEXT-TO-SPEECH | *Hieu-Thi Luong1,2, Junichi Yamagishi**1,2,3* | 1SOKENDAI (The Graduate University for Advanced Studies), Kanagawa, Japan 2National Institute of Informatics, Tokyo, Japan 3The University of Edinburgh, Edinburgh, UK | Bootstrap a VC system from a pretrained speaker-adaptive TTS model and unify the techniques as well as the interpretations of these two tasks. Our subjective evaluations show that the proposed framework is able to not only achieve competitive performance in the standard intra-language scenario but also adapt and convert using speech utterances in an unseen language. | voice conversion, cross-lingual, speaker adaptation, transfer learning, text-to-speech | https://export.arxiv.org/pdf/1909.06532 |
5 | WAVENET FACTORIZATION WITH SINGULAR VALUE DECOMPOSITION FOR VOICE | Hongqiang Du1,2, Xiaohai Tian2, Lei Xie*1*****, Haizhou Li******2*** | 1School of Computer Science, Northwestern Polytechnical University, xi’an, China 2Department of Electrical and Computer Engineering, National University of Singapore, Singapore hongqiang.du@u.nus.edu, eletia@nus.edu.sg, lxie@nwpu.edu.cn, haizhou.li@nus.edu.sg | We propose to use singular value decomposition (SVD) to reduce WaveNet parame- ters while maintaining its output voice quality. Specifically, we apply SVD on dilated convolution layers, and impose semi-orthogonal constraint to improve the performance. | Voice Conversion (VC), WaveNet, Sin- gular Value Decomposition (SVD) | http://lxie.nwpu-aslp.org/papers/2019ASRU_DHQ.pdf |
Paper 1: MIMO-SPEECH: END-TO-END MULTI-CHANNEL MULTI-SPEAKER SPEECH RECOGNITION
MIMO-Speech:端到端多通道多说话人语音识别(ASRU 2019 Best paper)https://arxiv.org/pdf/1910.06522.pdf
感想
ASRU的best paper思路是挺清晰的,但是与其他会议发表的classic论文相比还是感觉有一些些差距,有一些模型的细节点来说,会感觉有些晦涩难懂,打分:🌟🌟🌟
Abstract
MIMO-Speech,采用“多通道-输入”和“多通道-输出”,以建模 多通道 多说话人 情景下的语音分离和语音识别。
- 模型结构由三部分组成:
1)单声道masking网络;
2)多源神经波束形成器;
3)多输出-语音识别模型;
学习策略:a curriculum learning strategy
实验结果:60% WER reduction
Introduction (1 page)
待解决问题:鸡尾酒聚会课题,分为 单通道 / 多通道 语音识别问题
单通道多说话人语音分离:
1) Deep clustering (DPCL), 将时域单元映射到嵌入向量,再采用聚类算法将每个单元聚类到说话人源。此方法之后被嵌入到端到端训练框架中。
2)Permutation-invariant training (PIT):用一个permutation-free目标函数来最小化重构损失。PIT之后被应用于多说话人ASR,在一个DNN-HMM混合ASR框架下。
多通道多说话人语音分离:
1)PIT,语音分离
2)unmixing transducer (a mask-based beamformer),语音分离
3)DPCL:将inter-channel differences作为空间特征,和单通道频谱特征,语音分离
本文贡献:多通道-多说话人-语音识别,输入multi-channel input (MI), 输出 multiple output (MO) text sequences, one for each speaker, 所以称为 MIMO-Speech。
可行性:最近的单说话人-远场-语音识别展示了 “神经波束”技巧对于去噪的价值,并且一些研究证实了end-to-end的可行性。[27] 进一步证实了神经波束方法在多通道端到端系统能够增强信号。
MIMO-Speech (2 pages)
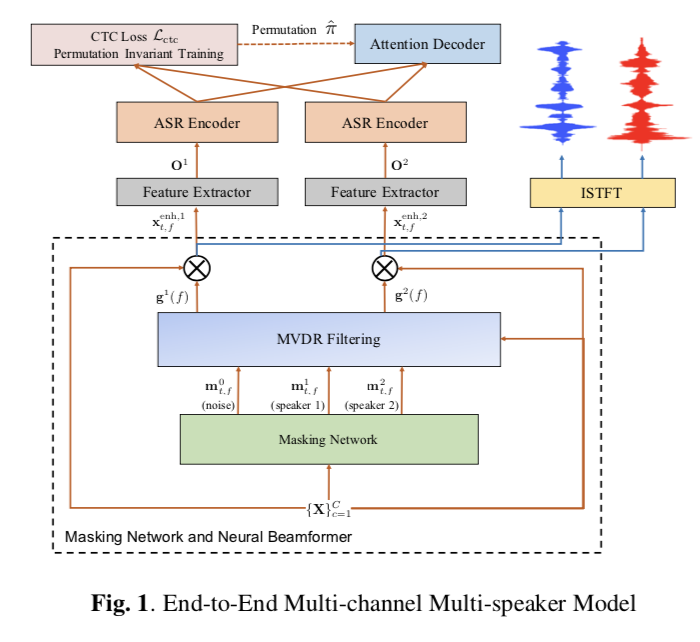
MIMO-Speech
Model architecture
- Stage 1: a single-channel masking network,通过预测多说话人和各通道噪音masks来实现预分离
- Stage 2: 多源 “神经波束” 来空间上分离多说话人的源头
- Stage 3: 端到端ASR来实现多说话人语音识别
创新点:masking network + neural beamformer,单目标函数进行模型训练。
Stage1 (Monaural masking network) 可以预分离开噪音和多说话人音源;Stage 2 生成多个beamforming filters \(g^{i}(f)\) 用来分离和降噪输入的多声道信号;Stage3有多个说话人的encoder,和一个attention decoder组成,来生成多个说话人的文本序列输出。
Data scheduling and curriculum learning
问题痛点:端到端训练难以收敛
解决方案(Data scheduling):随机从以下两个数据集中选择一个batch
1) 不仅采用多空间域的多说话人数据集
2) 也采用单说话人的数据集
- 细节(Curriculum learning) 配Algorithm:
1) 当选择到单说话人的数据集的时候,数据不经过 masking network 和 neural beamformer 模型,以加强end-to-end ASR模型的训练。
2) 计算出最大声和最小声说话人声音之间的信噪比SNR,然后按“升序”排列,从SNR=1的数据集开始训练
3) 将单说话人的数据集从短到长进行排序,让seq2seq模型首先学习短语句。
Experiments (3 pages)
3.1 Configurations
3.1.1 Neural Beamformer
3.1.2 Encoder-Decoder Network
3.2 Performance on ASR
Motivation: 验证提出的模型好于baselines
Baselines / Ours
3.3 Performance on Speech seperation
Motivation: 验证 neural beamformer 学习了一个波束行为,能够用于语音分离。
3.4 Evaluation on spatialized reverberant data (在空间混响数据上的实验)
Motivation: 验证在实际情况下的模型性能。
Paper 2: IMPROVING MANDARIN END-TO-END SPEECH SYNTHESIS BY SELF-ATTENTION AND LEARNABLE GAUSSIAN BIAS
Paper 2: 通过自注意力机制和学习高斯bias来提升中文普通话端到端语音合成系统 http://lxie.nwpu-aslp.org/papers/2019ASRU_YFY.pdf
感想
有些论文读起来会觉得高深莫测,但是有有部分价值可以吸取,打分:🌟🌟
Abstract
问题痛点:虽然对于英文来讲,现有的如Tacotron等模型已经能够实现端到端的语音合成过程,即从英文字母直接转换至语音。但是对于如中文这样的语言,仍旧需要繁复的前处理过程(如词边界、韵律边界等),使得这个文本处理前端的过程和传统方法一样复杂。
解决方案:为了保持生成语音的自然度、以及摒弃特定语言的特殊性,普通话语音合成过程中,我们引入了一个创新性的自注意力机制作为编码器,并且引入可学习的高斯bias到Tacotron中
实验结果:我们评估了不同的系统(在 有/无 韵律信息的情况下),结果显示提出的方法能够在最小的语言-依赖的前端模块的情况下,生成稳定和自然的语音。
Introduction (1页)
- 待解决问题
传统的端到端方法包含复杂的特征提取过程,如:Part-of-speech tagging, pronunciation prediction, prosody labelling. 即便如Tacotron的端到端语音合成系统被提出,但是单纯的输入音素也无法使得语音合成模型得到良好的效果,所以学者提出嵌入PW、PPH、IPH,来进行韵律边界的特征建模。但是这使得违背了端到端语音合成的初衷,使得这个系统再次变得更加复杂。
- 本文贡献
1)全局韵律建模:由于self-attention被证明对于简单的音素序列进行全局建模时有良好的效果,所以本文尝试采用自-注意力机制作为编码器来获取全局的韵律信息。
2)局部韵律建模:至于局部的韵律信息,我们采用了一个可学习的高斯bias引入到自注意力机制中,因为Gaussian分布更加集中于当前位置的局部关系。
Proposed SAG-Tacotron (1.5页)
3.1 Motivation
目的:为了采用最少的文本分析模块,所以引入自-注意力学习机制,来进行全局依赖建模。
方案:1)将Encoder的CBHG模块,用自-注意力机制替换;2)可学习的高斯bias来提升局部建模。
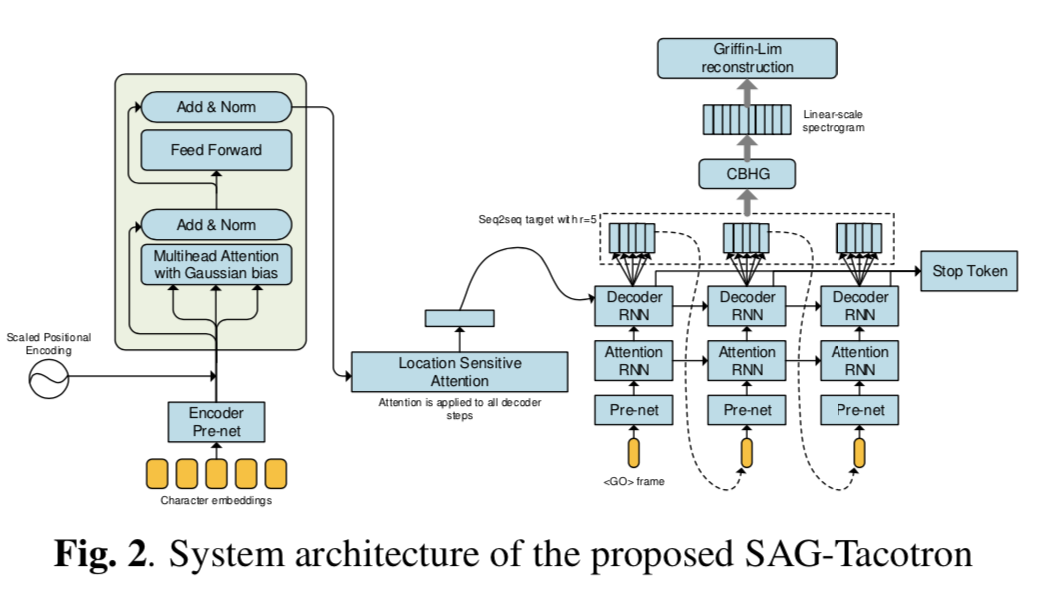
SAG-Tacotron
3.2 基于 自注意力机制 的 编码器
Encoder 的 Pre-net是一个3层-CNN + Batch Norm + ReLU,尽管自-注意力机制不包含序列信息,我们注入类似于Transformer的位置信息。 \[ PE_{pos,2i}=sin(pos/10000^{2i/d}) \]
\[ PE_{pos, 2i+1} = cos(pos/10000^{2i/d}) \]
其中,\(pos\)是当前位置,\(d\)是特征维度,\(i\)是当前维度。PE也被输入到自-注意力模块。自注意力模块包含了一个自注意力层+全连接层+tanh激活函数。残差连接被应用于上述层。
对于多头注意力机制的每一个\(head_i\), 对于一个有\(n\)个元素的序列\(x\),我们想要获得有相同长度n的隐状态向量\(head_i\), 这里采用scale-product注意力机制。 \[ Head_{i}=\sum_{j=1}^{n}ATT(Q,K)V \]
\[ ATT(Q, K)=softmax(energy) \]
\[ energy = \frac {QK^{T}} {\sqrt{d}} \]
最终的多头注意力机制为: \[ MultiHead(Q,K,V)=Concat(head_{1}, ..., head_{h})W^{O} \] 其中的\(W^{O}\)是最后一层线性层的参数矩阵。
3.3 可学习的Gaussian bias
在序列-序列模型中,对于中文来讲,相近的位置是十分重要的。在这种情况下,我们想要为注意力机制提升编码器对于临近状态的局部贡献。
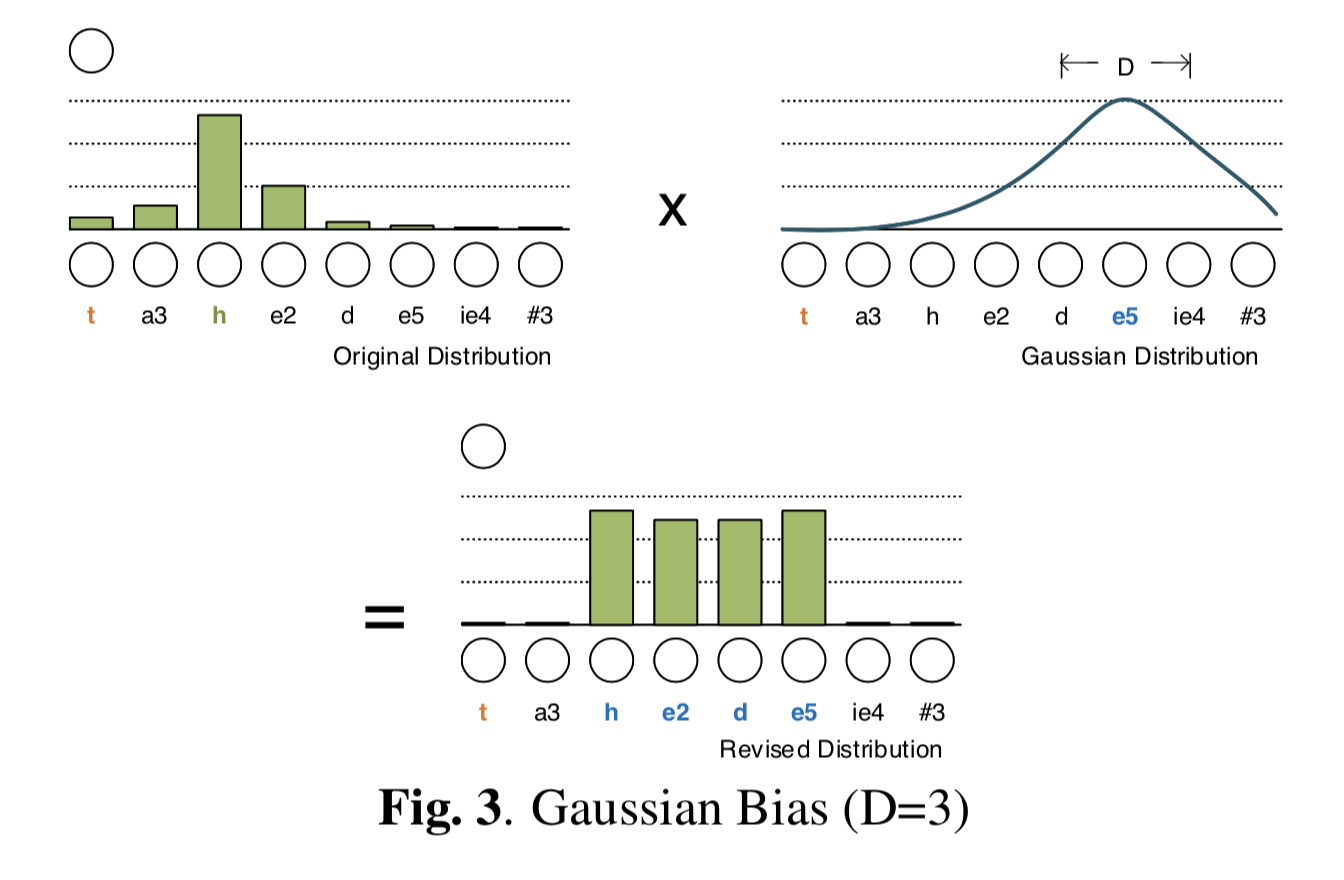
Gaussian-bias
如上图所示,首先,假设一个以e5为中心的高斯bias,窗长为3(实际上,窗长是一个可学习的参数)。然后将注意力机制的分布通过高斯bias来进行正则化,以生成最终的分布。如图3所示,最终的分布是会在e5附近有更多的权重的。
具体来讲,Gaussian bias \(G\)被mask到energy上,即 \[ ATT(Q, K)=softmax(energy + G) \]
其中\(G \in R^{N\times N}\),\(G \in (-1;0]\) 度量了当前的query \(x_i\)与position \(j\) 之间的关系: \[ G_{ij}=-\frac {(j - P_{i})^2}{2\sigma_i^2} \]
其中的\(P_i\)是\(x_i\)的中心位置,当给定输入序列 \(x=(x_1, x_2, ..., x_n)\),\(\sigma_i\)是标准差。如何选择合适的\(P_i\)和\(\sigma_i\)是关键。 \[ P_i = N\cdot sigmoid(v_p^{T}tanh(W_{p}x_i)) \]
\[ D_i = N\cdot sigmoid(v_d^{T}tanh(W_{d}x_i)) \]
其中\(\sigma_i = \frac {D_i}{2}\), \(W_p\) 和\(W_d\)是模型参数矩阵。
Experiments (2.5页)
4.1 Basic setups
4.2 System comparison
- Baseline: Tacotron1
- Baseline-prosody: Tacotron1 with complex inputs
- SAE-Tacotron: Self-attention as encoder without Gaussian bias with simply inputs
- SAG-Tacotron: Self-attention as encoder with Gaussian bias with simple inputs
- Transformer with simple inputs

simple_inputs
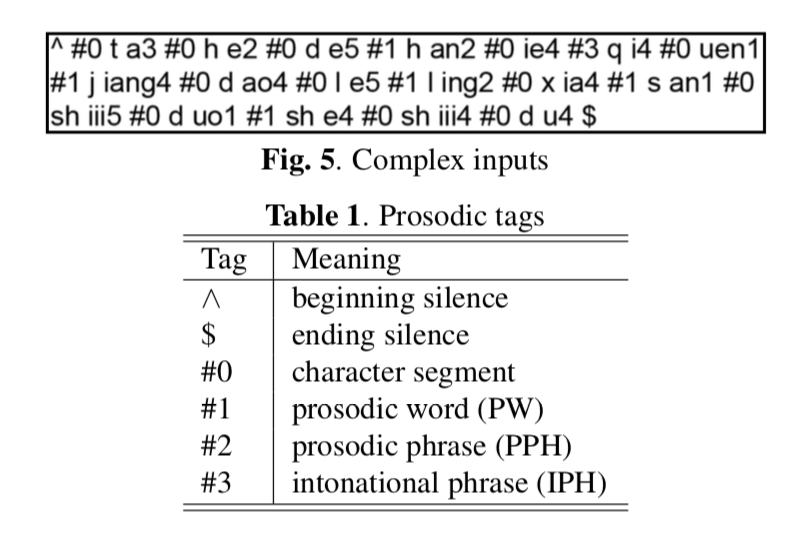
complex_inputs
4.3 Model details
4.4 Results
4.4.1 Robustness test
Motivation: 评估attention对齐(Repeats / Skips)的鲁棒性
4.4.2 Prosody analysis
Motivation: 评估重读音节的pitch以及trajectory pattern of F0
4.4.3 Objective test
Motivation: 采用MCD评估学习到的频谱的质量,MCD越低越好。
4.4.4 Subjective test
Motivation: 评估模型主管听测效果
评估方式:20个人,30句随机抽取的语音